Women still less likely to be hired, promoted, mentored or even have their research cited
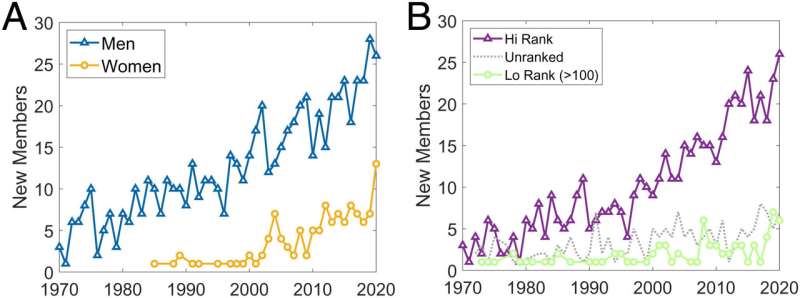
It’s 2022 and women in science are still less likely than their male peers to be hired and promoted. Women are less likely to be mentored by eminent faculty, they publish in less prestigious journals, have fewer collaborators, are underrepresented among journal reviewers and editors, and their papers receive fewer citations. How is this happening?
USC’s Information Sciences Institute (ISI) Principal Scientist Kristina Lerman and her team used AI to look for answers to this question. The resulting paper has been published in the peer-reviewed, multi-disciplinary science journal Proceedings of the National Academy of Sciences (PNAS) on September 26, 2022.
As a woman in science herself, Lerman knows the world she works in, but even she was shocked by statistics she recently learned: only 2% of Nobel Prize winners in physics have been women (until a few years ago that was 1%) and those numbers are similar across many scientific fields. Lerman said, “only 7% of Nobel Prize winners in chemistry have been women! Women have been working in chemistry for such a long time, so how is that? We were curious about this discrepancy.”
Right data, right time
Lerman had the right dataset for the problem. Since 2019, she and her team had been working on a large project that used AI to predict the reproducibility of research papers. The ISI team used AI to analyze many aspects of scientific papers, including the citations, to predict reproducibility. They published the paper “Assessing Scientific Research Papers with Knowledge Graphs” at ACM SIGIR 22 (the Association for Computing Machinery’s Special Interest Group on Information Retrieval) in July 2022, describing their novel method and promising findings.
To do this reproducibility research, Lerman’s team gathered a huge amount of data on academic papers. Her co-author Jay Pujara, director of the Center on Knowledge Graphs at ISI said, “We collected this very large citation graph—the network of papers, authors, citations, references, collaborations, author institutions, where they publish, etc.” They turned this data into a vast knowledge graph (a “knowledge graph” is a representation of a network of real-world entities that illustrates the relationships between them).
The team looked at the shapes or “structures” that arose in the knowledge graph. They wondered if there was some kind of natural phenomenon causing the different structures in the citation networks. Additionally, they wanted to make sure that the data used in their reproducibility predictions was not being impacted by biases in the data. Pujara said, “Kristina [Lerman] had the idea to look at covariates like gender or prestige.” And with that idea, the team of researchers set out to see if there was a difference in a network based on whether the author was a man or a woman, as well as if they were at a top ranked university or a lower ranked university.
The who, what and why of citations
Before we go any further, a little info on how citation in scientific research works. There are typically three reasons an author might cite another author’s paper.
First, as background—in order to understand their paper, an author will cite other papers that give the background information needed. Second, to explain a method—if an author used a method that’s similar, a version of, or comparable to a method from another paper they will cite the paper that explains that method. And third, results—an author will explain their results, but might cite other papers that studied that same thing but got different results.
Gleaning information from citations
“Trying to study the citation network for every researcher out there is really hard, so why don’t we pick the cream of the crop?” said Pujara. The team looked at scientists elected to the U.S. National Academy of Sciences (NAS), one of the oldest and most prominent professional science organizations. New members of NAS are elected by current members based on a distinguished record of scientific achievement meaning, in theory, they’ve all reached the same echelon of recognition. The ISI team looked at 766 NAS researchers, 120 of whom were women, hypothesizing that complex gender differences would be visible within this group of elite scientists.
Their hypothesis proved correct.
They constructed citation networks that captured the structure of peer recognition for each NAS member. These structures differed significantly between male and female NAS members. Women’s networks were much more tightly clustered, indicating that a female scientist must be more socially embedded and have a stronger support network than her male counterparts. The differences were systemic enough to allow the gender of the member to be accurately classified based on their citation network alone.
Lerman said, “We could write an AI algorithm that would just look at the citation networks and predict whether this was the citation network of a woman or a man. This was pretty shocking and disappointing to us.”
As a control study, the team also looked at the covariate of prestige. NAS members affiliated with less prestigious institutions are a minority in NAS, similar to women. Lerman said, “we would have imagined that maybe women’s citation networks would look like those of members from non-prestigious universities.” But that was not the case. They did not observe any disparities due to the prestige of a member’s institutional affiliation.
Conclusion: based on a scientist’s citation network alone, gender can accurately be determined, but the prestige of the university that scientist is affiliated with cannot. This suggests that gender continues to influence career success in science, according to the ISI team.
How to stop being so short-cited
Why is this happening? Pujara said, “We don’t know. It could be because there’s some aspect of gender that changes collaborative behavior. Or it might be something about society that shapes researchers and their paths based on social biases. So we don’t actually know the answer to that. What we know is that there’s a difference.”
The real question is: how can we change it? How can we make science a less hostile climate for women, remove the barriers to opportunities for women, and create an environment that allows women to rise to the top of their fields?
The ISI team hopes that, moving forward, their methods and results can help. To start, this study could be used to help researchers understand what their networks look like. Additionally, it could be used as a way for policymakers to understand if programs aiming to improve gender equity in science are working.
Finally, and importantly, we can learn from those differences in the citation structures between men and women. “For a woman to be recognized, she has to be well-embedded and have a strong support network,” Lerman said. “Mentoring young women and telling them they really have to build those networks of social support, and be very intentional about them” seems to be one way to change the shape of these structures… and the shape of science.
Researchers find citation bias in published papers and evidence that the problem is getting worse
Kristina Lerman et al, Gendered citation patterns among the scientific elite, Proceedings of the National Academy of Sciences (2022). DOI: 10.1073/pnas.2206070119
Citation:
Women still less likely to be hired, promoted, mentored or even have their research cited (2022, September 27)
retrieved 27 September 2022
from https://phys.org/news/2022-09-women-hired-cited.html
This document is subject to copyright. Apart from any fair dealing for the purpose of private study or research, no
part may be reproduced without the written permission. The content is provided for information purposes only.
For all the latest Science News Click Here
For the latest news and updates, follow us on Google News.