Simulations with a machine learning model predict a new phase of solid hydrogen
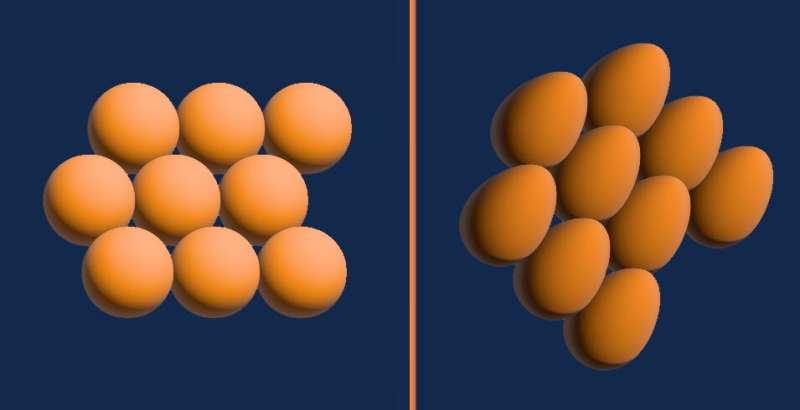
Hydrogen, the most abundant element in the universe, is found everywhere from the dust filling most of outer space to the cores of stars to many substances here on Earth. This would be reason enough to study hydrogen, but its individual atoms are also the simplest of any element with just one proton and one electron. For David Ceperley, a professor of physics at the University of Illinois Urbana-Champaign, this makes hydrogen the natural starting point for formulating and testing theories of matter.
Ceperley, also a member of the Illinois Quantum Information Science and Technology Center, uses computer simulations to study how hydrogen atoms interact and combine to form different phases of matter like solids, liquids, and gases. However, a true understanding of these phenomena requires quantum mechanics, and quantum mechanical simulations are costly. To simplify the task, Ceperley and his collaborators developed a machine learning technique that allows quantum mechanical simulations to be performed with an unprecedented number of atoms. They reported in Physical Review Letters that their method found a new kind of high-pressure solid hydrogen that past theory and experiments missed.
“Machine learning turned out to teach us a great deal,” Ceperley said. “We had been seeing signs of new behavior in our previous simulations, but we didn’t trust them because we could only accommodate small numbers of atoms. With our machine learning model, we could take full advantage of the most accurate methods and see what’s really going on.”
Hydrogen atoms form a quantum mechanical system, but capturing their full quantum behavior is very difficult even on computers. A state-of-the-art technique like quantum Monte Carlo (QMC) can feasibly simulate hundreds of atoms, while understanding large-scale phase behaviors requires simulating thousands of atoms over long periods of time.
To make QMC more versatile, two former graduate students, Hongwei Niu and Yubo Yang, developed a machine learning model trained with QMC simulations capable of accommodating many more atoms than QMC by itself. They then used the model with postdoctoral research associate Scott Jensen to study how the solid phase of hydrogen that forms at very high pressures melts.
The three of them were surveying different temperatures and pressures to form a complete picture when they noticed something unusual in the solid phase. While the molecules in solid hydrogen are normally close-to-spherical and form a configuration called hexagonal close packed—Ceperley compared it to stacked oranges—the researchers observed a phase where the molecules become oblong figures—Ceperley described them as egg-like.
“We started with the not-too-ambitious goal of refining the theory of something we know about,” Jensen recalled. “Unfortunately, or perhaps fortunately, it was more interesting than that. There was this new behavior showing up. In fact, it was the dominant behavior at high temperatures and pressures, something there was no hint of in older theory.”
To verify their results, the researchers trained their machine learning model with data from density functional theory, a widely used technique that is less accurate than QMC but can accommodate many more atoms. They found that the simplified machine learning model perfectly reproduced the results of standard theory. The researchers concluded that their large-scale, machine learning-assisted QMC simulations can account for effects and make predictions that standard techniques cannot.
This work has started a conversation between Ceperley’s collaborators and some experimentalists. High-pressure measurements of hydrogen are difficult to perform, so experimental results are limited. The new prediction has inspired some groups to revisit the problem and more carefully explore hydrogen’s behavior under extreme conditions.
Ceperley noted that understanding hydrogen under high temperatures and pressures will enhance our understanding of Jupiter and Saturn, gaseous planets primarily made of hydrogen. Jensen added that hydrogen’s “simplicity” makes the substance important to study. “We want to understand everything, so we should start with systems that we can attack,” he said. “Hydrogen is simple, so it’s worth knowing that we can deal with it.”
More information:
Hongwei Niu et al, Stable Solid Molecular Hydrogen above 900 K from a Machine-Learned Potential Trained with Diffusion Quantum Monte Carlo, Physical Review Letters (2023). DOI: 10.1103/PhysRevLett.130.076102
Citation:
Simulations with a machine learning model predict a new phase of solid hydrogen (2023, April 22)
retrieved 22 April 2023
from https://phys.org/news/2023-04-simulations-machine-phase-solid-hydrogen.html
This document is subject to copyright. Apart from any fair dealing for the purpose of private study or research, no
part may be reproduced without the written permission. The content is provided for information purposes only.
For all the latest Science News Click Here
For the latest news and updates, follow us on Google News.