Researchers propose a deep neural network-based 4-quadrant analog sun sensor calibration
by Beijing Institute of Technology Press Co.
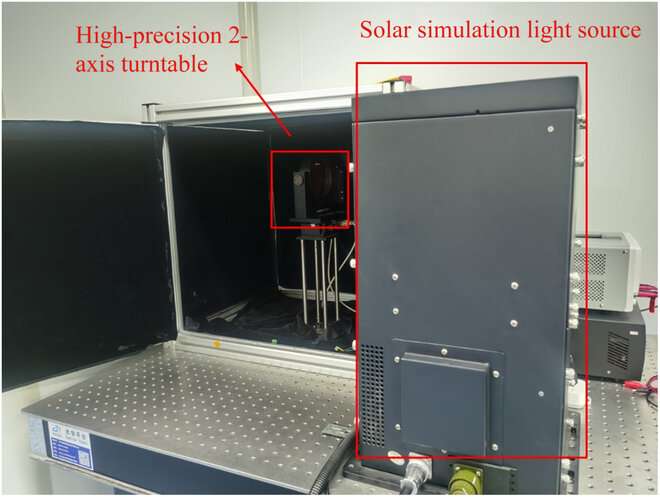
A spacecraft can estimate the attitude state by comparing external measurements from attitude sensors with reference information. CubeSats tend to use 4-quadrant analog solar sensors which have the advantages of extremely low power consumption, minimal volume, low complexity, low cost, and high reliability as attitude sensors, considering the limitation of satellite volume and payload. The performance of the sensor can be importantly improved by the calibration procedure and compensation model.
However, the various error sources affecting the calibration of the 4-quadrant sun sensor lead to a complicated process of compensation model establishment. Deep learning, which is widely used in the aerospace field in recent years, is able to approximate any continuous function on a bounded closed set, providing new ideas for solving the traditional problem.
In a research paper recently published in Space: Science & Technology, authors from Northwestern Polytechnical University, German Aerospace Center, and Dalian University of Technology together propose a method to calibrate sun sensors by deep learning, which not only is able to integrate the influence of various errors but also avoids the need of analyzing and modeling every single error.
Authors first explain the calibration process of sun sensor based on cubic surface fitting. The calibration and testing platform of the sun sensor includes a solar simulation light source, high-precision 2-axis turntable, and servo controller. The outputs of the sun sensor are the incidence λ and azimuth υ, whose theoretical values are obtained from the angle of the turntable and actual values are measured by the sun sensor. There are large errors between the theoretical and actual values, up to 3°.
By substituting the theoretical and actual values into the 3-order surface fitting formula, the cubic surface fitting model are obtained. The output value of the analog sun sensor is corrected by the cubic surface fitting model and the error is effectively reduced. However, there’re larger errors close to the edge of FOV (field of view) of the sun sensor.
Then, authors introduce the learning and training of the deep neural network model, which approximates the actual error model and is used for error correction. In the calibration experiment of the analog sun sensor, the deep feedforward neural network is selected to fit the error model, where ReLU (linear rectification function) is selected as the activation function, a full connection as the connection mode between 2 adjacent layers, and the mean square error (MSE) as the loss function. The neural network is trained through gradient descent algorithm and the backpropagation algorithm.
Finally, the effectiveness of the proposed algorithm is verified using experimental data. In the calibration experiment of an analog sun sensor, the training of the network model is divided into two stages: the dataset is generated by the cubic surface fitting model in the initial training stage while the deep network model adopts the measured data for learning in the final training stage.
Additionally, learning data are normalized to the magnitude between 0 and 1 via the max–min method. After network learning in the initial stage, the error between the output of the network model and the estimated value of the cubic surface fitting model is concentrated within 0.02°, indicating that the deep network model at this time can better map the cubic surface fitting model.
In the final training stage of the feedforward neural network model, the calibration error of the incident angle can reach 0.1° (1σ) and 0.25° (3σ), which greatly improves the calibration effect of the cubic surface fitting model. These results shows that the method can effectively eliminate deterministic errors, including spot distortion and assembly errors.
More information:
Qinbo Sun et al, Deep Neural Network-Based 4-Quadrant Analog Sun Sensor Calibration, Space: Science & Technology (2023). DOI: 10.34133/space.0024
Provided by
Beijing Institute of Technology Press Co.
Citation:
Researchers propose a deep neural network-based 4-quadrant analog sun sensor calibration (2023, May 30)
retrieved 30 May 2023
from https://phys.org/news/2023-05-deep-neural-network-based-quadrant-analog.html
This document is subject to copyright. Apart from any fair dealing for the purpose of private study or research, no
part may be reproduced without the written permission. The content is provided for information purposes only.
For all the latest Science News Click Here
For the latest news and updates, follow us on Google News.