How scientists predict solar wind speed accurately using multimodality information
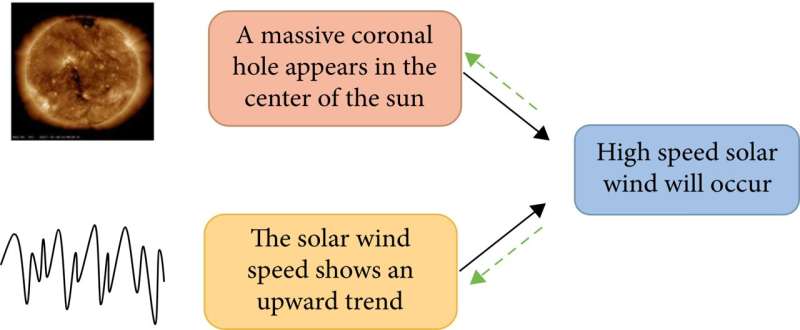
As more and more high-tech systems are exposed to the space environment, space weather prediction can provide better protection for these devices. In the solar system, space weather is mainly influenced by solar wind conditions. The solar wind is a stream of supersonic plasma-charged particles which will cause geomagnetic storms, affect short-wave communications, and threaten the safety of electricity and oil infrastructure when passing over the Earth.
Accurate prediction of the solar wind speed will allow people to make adequate preparations to avoid wasting resources. Most existing methods only use single-modality data as input and do not consider the information complementarity between different modalities. In a research paper recently published in Space: Science & Technology, Zongxia Xie, from College of Intelligence and Computing, Tianjin University, proposed a multimodality prediction (MMP) method that jointly learnt vision and sequence information in a unified end-to-end framework for solar wind speed prediction.
First, the author introduced the overall structure of MMP, which includes a vision feature extractor, Vmodule, and a time series encoder, Tmodule, as well as a Fusion module. Next, the structures of Vmodule and Tmodule were introduced. Image data and sequence data were processed by Vmodule and Tmodule, respectively. Vmodule used the pretrained GoogLeNet model as a feature extractor to extract Extreme Ultraviolet (EUV) image features.
Tmodule consisted of a convolutional neural network (CNN) and a bidirectional long short-term memory (BiLSTM) to encode sequence data features for assisting prediction. A multimodality fusion predictor was included, allowing feature fusion and prediction regression. After extracting features from two modules, the two feature vectors were concatenated into one vector for multimodality fusion. The prediction results were obtained by a multimodality prediction regressor. The multimodality fusion method was applied to realize information complementary to improve the overall performance.
Then, to verify the effectiveness of the MMP model, the author conducted some experiments. The EUV images observed by the solar dynamics observatory (SDO) satellite and the OMNIWEB dataset measured at Lagrangian point 1 (L1) were adopted to the experiment. The author preprocessed EUV images and the solar wind data from 2011 to 2017.
Since time series data had continuity in the time dimension, the author split data from 2011 to 2015 as the training set, data from 2016 as the validation set, and 2017 as the test set. Afterwards, the experimental setup was described. The author finetuned the GoogLeNet pretrained on the ImageNet dataset to extract EUV image features.
Metrics such as Root Mean Square Error (RMSE), Mean absolute error (MAE), and Correlation Coefficient (CORR) were used for comparison to evaluate the continuous prediction performance of the model. RMSE was calculated by taking the square root of the arithmetic mean of the difference between the observed value and the predicted value.
MAE represented the mean of absolute error between the predicted and observed value. CORR can represent the similarity between the observed and the predicted sequence. Moreover, the Heidke skill score was adopted to evaluate whether the model can capture the peak solar wind speed accurately.
Comparative experiments showed that MMP achieves best performance in many metrics. Besides, to prove the effectiveness of each module in the MMP model, the author conducted ablation experiments. It could be seen that removing the Vmodule led to a decline in experimental results, especially for long-term prediction. In contrast to the removal of Vmodule, removing Tmodule had a more significant impact on short-term prediction.
The author also compared the performance of different pretrained models to verify the effectiveness of them to capture image features and found out that GoogLeNet obtained the most and the best metric results. Moreover, hyperparameter comparison experiments were conducted to verify the rationality of our model parameter selection.
Finally, the author proposed several promising directions for the future work. Firstly, future research would focus on the impact of different modalities on performance, assign different weights to different modalities, and use their complementary relationship to improve performance. Secondly, the proposed model cannot capture high-speed solar stream well, which was very difficult but essential for the application. Thus, the author would focus on how to improve peak prediction in the future.
Yanru Sun et al, Accurate Solar Wind Speed Prediction with Multimodality Information, Space: Science & Technology (2022). DOI: 10.34133/2022/9805707
Provided by
Beijing Institute of Technology Press Co., Ltd
Citation:
How scientists predict solar wind speed accurately using multimodality information (2022, October 18)
retrieved 19 October 2022
from https://phys.org/news/2022-10-scientists-solar-accurately-multimodality.html
This document is subject to copyright. Apart from any fair dealing for the purpose of private study or research, no
part may be reproduced without the written permission. The content is provided for information purposes only.
For all the latest Science News Click Here
For the latest news and updates, follow us on Google News.