Deep learning solves long-standing challenges in identification of nanoparticle shape
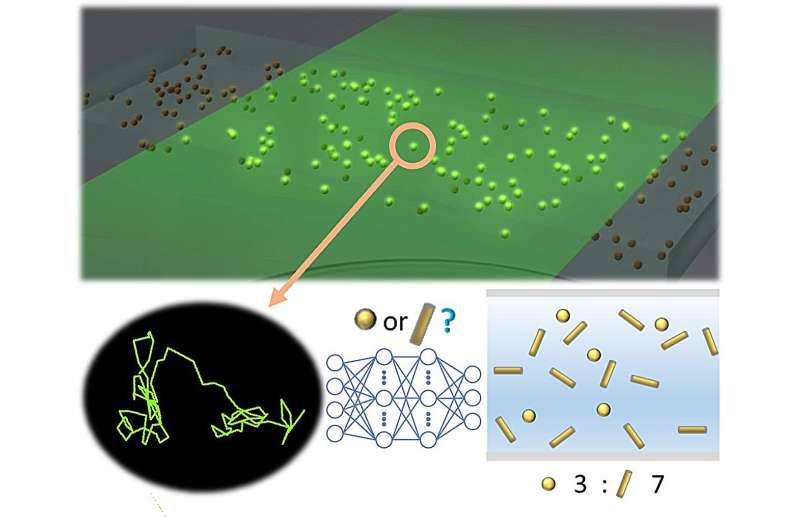
Innovation Center of NanoMedicine has announced with The University of Tokyo that a group led by Prof. Takanori Ichiki, Research Director of iCONM, proposed a new property evaluation method of nanoparticles’ shape anisotropy that solves long-standing issues in nanoparticle evaluation that date back to Einstein’s time.
The paper, titled “Analysis of Brownian motion trajectories of non-spherical nanoparticles using deep learning” was published online in APL Machine Learning.
In this era where new medical treatments and diagnostic technologies using extracellular vesicles and artificial nanoparticles are attracting attention, nanoparticles are useful materials in the medical, pharmaceutical, and industrial fields. From a materials perspective, it is necessary to evaluate the properties and agglomeration state of each nanoparticle and perform quality control, and progress is expected in nanoparticle evaluation technology that supports safety and reliability.
One way to evaluate nanoparticles in liquid is to analyze the trajectory of Brownian motion. Called NTA, it calculates the diameter of a particle using a theoretical formula discovered by Einstein over 100 years ago. Although it is used as a simple method to measure single particles from micro to nano size, there has been a long-standing problem that it cannot evaluate the shape of nanoparticles.
The trajectory of Brownian motion reflects the influence of particle shape, but it is difficult to actually measure extremely fast motion. Furthermore, even if the particle is non-spherical, conventional analysis methods are not accurate because they unconditionally assume that the particle is spherical and use the Stokes-Einstein equation for analysis.
However, using deep learning, which is good at finding hidden correlations in large-scale data, it is possible to detect differences caused by differences in shape may be detected, even when measurement data is averaged or contains errors that cannot be separated.
Prof. Takanori Ichiki’s research group succeeded in building a deep learning model that identifies shapes from measured Brownian motion trajectory data without changing the experimental method. In order to take into account not only the time-series changes in data but also the correlation with the surrounding environment, they integrated a 1-dimensional CNN model that is good at extracting local features through convolution and a bidirectional LSTM model that can accumulate temporal dynamics.
Through trajectory analysis using the integrated model, they were able to achieve classification accuracy of approximately 80% on a single particle basis for two types of gold nanoparticles that are approximately the same size but have different shapes, which cannot be distinguished using conventional NTA alone.
Such high accuracy indicates that the shape classification of single nanoparticles in liquid using deep learning analysis has reached a practical level for the first time. Furthermore, in the paper, a calibration curve was created to determine the mixing ratio of a mixed solution of two types of nanoparticles (spherical and rod-shaped). Considering the shape types of nanoparticles available in the world, it is thought that this method can sufficiently detect the shape.
With conventional NTA methods, the particle shape cannot be directly observed, and the characteristic information obtained was limited. Although the trajectory of Brownian motion (time-series coordinate data) measured by the NTA device contains information on the shape of the nanoparticles, because the relaxation time is extremely short, it has been difficult to actually detect the shape anisotropy of nanoparticles. Furthermore, in conventional analysis methods, even if the particle is non-spherical, it is not accurate due to the shape factor not being applied, because it is assumed to be spherical and analyzed using the Stokes-Einstein equation.
The researchers aimed for a new method that anyone can implement, and were able to solve a long-standing problem in Brownian motion analysis by introducing deep learning, which is good at finding hidden correlations in large-scale data, into data analysis without changing simple experimental methods.
In this paper, they attempted to determine the shapes of two types of particles, but considering the types of shapes of commercially available nanoparticles, they think that this method can be used in practical applications such as the detection of foreign substances in homogeneous systems. Expansion of NTA will lead to applications not only in research but also in the industrial and industrial fields, such as evaluating the properties, agglomeration state, and uniformity of nanoparticles that are not necessarily spherical, and quality control.
In particular, it is expected to be a solution for evaluating the properties of diverse biological nanoparticles such as extracellular vesicles in an environment similar to that of living organisms. It also has the potential to be an innovative approach in fundamental research on Brownian motion of non-spherical particles in liquid.
More information:
Analysis of Brownian motion trajectories of non-spherical nanoparticles using deep learning, APL Machine Learning (2023). DOI: 10.1063/5.0160979
Provided by
Innovation Center of NanoMedicine
Citation:
Deep learning solves long-standing challenges in identification of nanoparticle shape (2023, October 24)
retrieved 24 October 2023
from https://phys.org/news/2023-10-deep-long-standing-identification-nanoparticle.html
This document is subject to copyright. Apart from any fair dealing for the purpose of private study or research, no
part may be reproduced without the written permission. The content is provided for information purposes only.
For all the latest Science News Click Here
For the latest news and updates, follow us on Google News.